AI Technologies Weren’t Born Yesterday
AI has been a source of speculation, observation (and entertainment) for more than a century
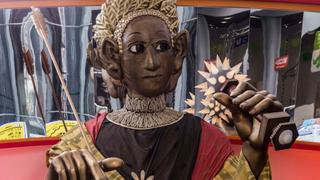
Those with any degree of following in media—social media and otherwise—have likely been trying to understand what this “new” technology known by the term “artificial intelligence” really means, where it was derived from, where it is headed and whether it yields another level of risk (human or otherwise).
In 1956, a small group of scientists gathered for the Dartmouth Summer Research Project on Artificial Intelligence—this is often said to be the birth of this field of research. Succeeding semiannual conferences on AI research have continued from that point forward.
Fifty years later, to celebrate its anniversary, more than 100 researchers and scholars again met at Dartmouth for AI@50, a conference formally known as the Dartmouth Artificial Intelligence Conference. This conference not only honored past and present accomplishments, but also helped seed ideas for future AI research.
Organizer John McCarthy, then a mathematics professor at the college, said the conference was “to proceed on the basis of the conjecture that every aspect of learning or any other feature of intelligence can in principle be so precisely described that a machine can be made to simulate it.”
Professor of Philosophy James Moor, the director of AI@50, said the researchers who came to Hanover, New Hampshire, 50 years ago thought about ways to make machines more cognizant and wanted to lay out a framework to better understand human intelligence.
Attendees of that workshop became the initial leaders of AI research for decades. Many predicted “machines as intelligent as humans would exist within a generation.”
100 Years Hence
Even before the Dartmouth AI Research projects, as early as the early 1900s, there was media created that centered around the idea of artificial humans. Scientists of all sorts began asking, “might it be possible to create an artificial brain? [sic]” Some creators even made some versions of what we now call “robots,” the word coined in a Czech play in circa 1921.
Most early robots were relatively simple, either steam- or pneumatic-powered for the most part. Some robots could make facial expressions and even walk. In 1955, the film “Forbidden Planet” featured the robot character with AI-like capabilities and by 1963, a “real” robot (played by Ray Walston) debuted in the CBS sitcom “My Favorite Martian” and anchored the idea of a more “human-like” robot with a personality and a purpose.
Between 1950 and the mid-1950s, interest in AI really came into being. Publications by scientists would create proposals that would consider the question, “Can machines think?” The first part of this consideration should begin by defining the meaning of the two terms “machine” and “think.” We note that this consideration is today commonly referred to as machine learning (ML)—a critical part of the primary element(s) in AI.
In the early years, AI definitions could have been framed to reflect the normal use of the words “artificial” and “intelligence,” but such a seemingly narrow attitude can be dangerous—a position we are facing today with the almost inexplicable use of the AI term being applied to anything and elevated to false impressions and/or mistrust.
Computer Intelligence: Machine ‘Learning’ or ‘Thinking?’
Philosophically, the meaning of the words “machine” and “think”—when connected or used together—make it difficult to avoid the conclusion that both the “meaning” and the “answer” to the question “can machines think?” are one in the same. Definitions should not be developed just by adding another question into the answer.
Current AI solutions are often developed by repeated sampling and analyzing closely related solutions, and then making adjustments to the testing results (via algorithms) in such a way that the solution is developed by “honing in” on (i.e., sharpening) an answer that will not result in relatively ambiguous words.
In Alan Turing’s work “Computer Machinery and Intelligence” (which eventually became known as “The Turing Test,” circa the 1950s), experts could begin to use the methodologies to measure computer intelligence. Given that computers were essentially construed to be intelligent, another term was suggested (machine learning) and adopted to make the phrase a bit less ambiguous while leaving the solution set robust enough to allow improvements on the intended conclusion without leaving the actual solution “ambiguous.”
From observations like these, the term “artificial intelligence” was coined and over time came into popular use. Today, if AI’s meaning is thrust into a search engine, one might find the answer to be “a set of technologies that enable computers to perform a variety of advanced functions”—ambiguous to some degree, but still relatively understandable.
The Imitation Game
In 1950, Alan Turing explained in “Computer Machinery and Intelligence” a proposed means to test machine intelligence through an artificial game called “The Imitation Game.” The game uses three players, each isolated from one another, one of whom is an interrogator. The interrogator attempts through questioning to find out the gender (male or female) of the other two players. Interrogation is done in written (textual) form only so that voices do not give away the obvious answer. Questions continue until a conclusion is drawn about who amongst the two players is who, i.e., who is male and who is female.
This kind of testing is not unlike what happens in a modern AI environment—whereby questions are posed and answers (“data”) collected until sufficient information can point to an appropriate conclusion (aka an “answer”) to the problem. In Turing’s game, which later became “The Turing Process,” the next step changes the players somewhat by replacing one of the two “human players” (say the woman) with a machine (“computer”). The computer is then charged with simulating the substituted female human player.
Now the interrogator is challenged to find questions which can be answered honestly and accurately by both the initial (male) human or substitute (female) computer—and then from the data collected, determine which is the computer by using sufficient data and developing a proper conclusion.
IBM and Checkers
If the computer can imitate the replaced (in this case female human) elements; then ultimately by demonstration this drives the answer to the other (initial) question “Can Machines Think?”—the computer becoming the artificially intelligent “female” player.
Historically, a computer scientist named Arthur Samuel starting around 1952 developed a program to play checkers, which is the first recognized computerized application to ever “learn” the game independently.
Samuel’s programs were played by performing a “lookahead search” from each current position, essentially using the data to intelligently “predict” and test the outcome for all the remaining steps which might—or would—be played out by the pair of opposing players. Samuel first wrote the checkers-playing program for the IBM 701 and had his first “learning program” completed in 1955, which was later demonstrated on television in 1956.
Rote learning (“rote” being a memorization technique that involves repeating information until it Is remembered) and other aspects of Samuel’s work strongly suggest the essential idea of temporal-difference learning—that the value of a state should equal the value of likely following states. Samuel created this “learning by generalization” procedure which is used to modify the parameters of the value function to approach a testable and useful conclusion to the end, without ambiguities.
The entire fundamental basis for AI (i.e., “generative AI”) is built from this very simple process. Today, generative AI can be used to solve problems and can now even create new content such as images, videos, text, music and audio.
When applied to other practical purposes, it can be used to reduce costs, personalize experiences, analyze risk mitigation and apply to sustainability. However, there are drawbacks, as in any technology. Shortcomings may be the results are not accurate, may be biased (per the slant put into the testing algorithms used), may violate privacy or may infringe on copyright.
We’ve covered a bit of ground on this historic introduction and methodology summary, and in the future, we’ll dive a bit deeper into risk and management of AI—especially when it is applied widespread and uncontrolled.
Get the TV Tech Newsletter
The professional video industry's #1 source for news, trends and product and tech information. Sign up below.
Karl Paulsen recently retired as a CTO and has regularly contributed to TV Tech on topics related to media, networking, workflow, cloud and systemization for the media and entertainment industry. He is a SMPTE Fellow with more than 50 years of engineering and managerial experience in commercial TV and radio broadcasting. For over 25 years he has written on featured topics in TV Tech magazine—penning the magazine’s Storage and Media Technologies and Cloudspotter’s Journal columns.