For Content Personalization, ‘Taste’ Is All in the Data
Recommendation engines increase viewership, interaction
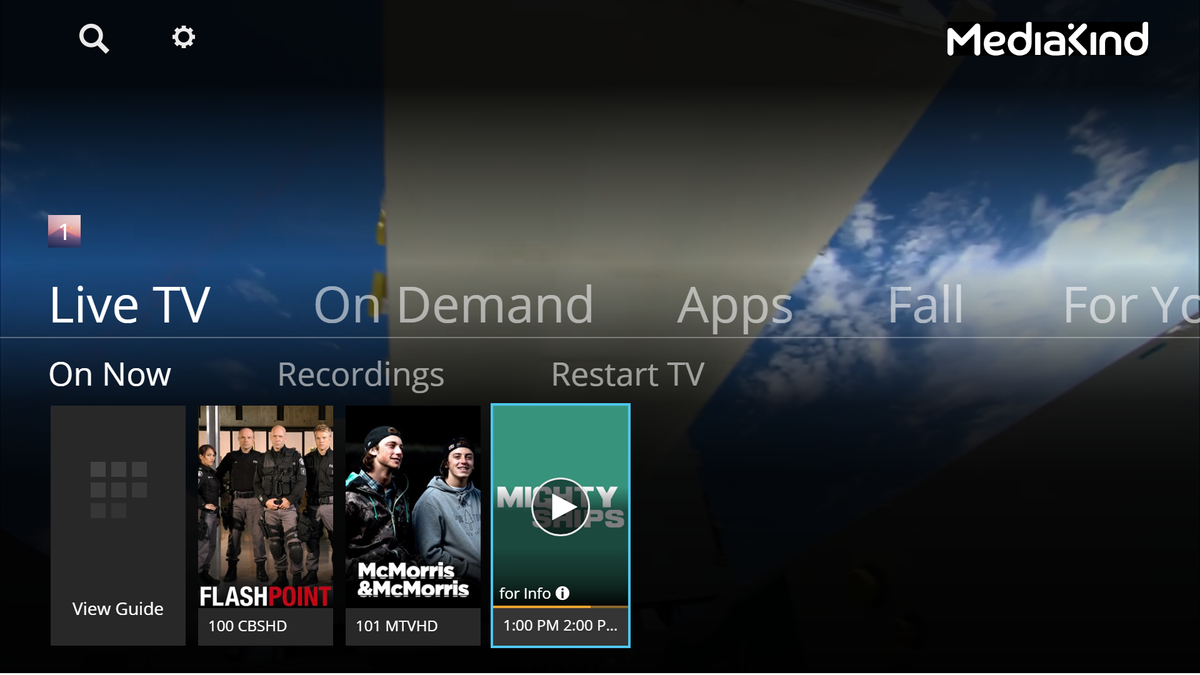
The applications may seem innocuous when they suggest new programs for you to watch, but content personalization/recommendation engines are truly powerful platforms that significantly benefit broadcasters and content distributors.
Case in point: ThinkAnalytics produces content personalization engines for Bell Canada, Crunchyroll, Tata, Liberty Global, SkyMexico and BritBox International, among others across 38 countries. Based on its presence on one billion devices serving 475 million people, ThinkAnalytics’ research has found that its content recommendations increase on-demand viewership up to 100%, boost the consumption of linear TV by 35% and increase the number of channels viewed by 30%.
“Content recommendation engines are all about placing the content in front of the subscriber in such a way that they’re going to act,” said Greg Riker, chief revenue officer with ThinkAnalytics in the U.K. “The key is making the viewing experience on that channel/platform interesting enough for them to continue to come back because they’re being served the content that they like to watch on an ongoing basis.”
How It Works
Content personalization is all about monitoring the types of content a given viewer chooses to watch—particularly when they do so for an entire series—and using the data accumulated during their viewing experiences to recommend similar kinds of content.
“There are two ways that you can use personalization,” said Tony Jones, principal technologist with MediaKind, which offers its MediaFirst TV recommendation platform. “First, there’s video on demand, where content appears as a recommendation for viewers based on their preferences. Second is the creation of a ‘Custom Linear Channel.’ This involves creating a custom channel filled with content from the recommendation engine. It’s like a continuous channel specifically crafted for an individual user, eliminating the need to swap channels. It’s essentially a recommendation-driven playlist.”
To do all of this successfully, “video content recommendation engines leverage several data sets to suggest content by analyzing a user’s interests and viewing behavior,” said JT Taylor, product marketing lead with Synamedia; maker of Synamedia Go, a cloud-based video management platform that includes content recommendation modules. “There are several factors that these recommendations are based on—for example, the viewer’s search and watch history, and the content they have previously liked or shared among others.
“By analyzing data, identifying patterns, and understanding viewer preferences, these engines, driven by algorithms developed with a combination of AI and research, offer personalized content recommendations that expand beyond surface level recommendations solely based on what someone has already watched,” Taylor continued. “They also enable viewers to discover new content that they might have otherwise overlooked.”
Get the TV Tech Newsletter
The professional video industry's #1 source for news, trends and product and tech information. Sign up below.
ThinkAnalytics refers to this concept as developing “taste profiles” that capture viewers’ program preferences in ways that can lead to successful content recommendations.
It’s an in-depth process: “We do a lot of what we call ‘metadata enrichment’ to get much more granular on the ability to make good recommendations,” said Riker. “It’s not just that you’re watching a drama or a romcom or that you like this actor. We get much more detailed than that. It’s the ability to compare that taste profile against the rest of the catalog and then put similar content in front of this person so they continue to watch this channel.”
As the numbers from ThinkAnalytics demonstrate, content personalization can help keep viewers watching the same channel/platform more often. This reduces churn at a time of ever-fragmenting audiences due to increased content choice.
Taylor concurs. “Ultimately, the synergy between personalized recommendations and content discovery increases user loyalty and leads to greater lifetime value for each user,” he said.
From ThinkAnalytics’ standpoint, it’s about engagement, according to Riker.
“That’s really important, he said. “If you can begin to offer similar content and advertising to viewers, you can keep their engagement levels very high, and then you can go from there.”
Privacy and Security Concerns
Although content personalization platforms do harvest viewership data, they do so in such a way that their identities remain anonymous and their personal information stays private.
“Privacy is paramount,” Jones said. “Personalization tools should ensure that individual users remain unidentified. A token-based system can ensure user or group anonymity. The design of personalization engines must prioritize the end consumer’s privacy.”
Striking a balance between offering personalized content recommendations and respecting user privacy is crucial to ensure customer trust and maintain a positive relationship with users.”
JT Taylor, Synamedia
His view is endorsed by others in the industry. “Striking a balance between offering personalized content recommendations and respecting user privacy is crucial to ensure customer trust and maintain a positive relationship with users,” said Taylor. “As a responsible technology provider, we prioritize implementing robust data protection measures and providing transparency to address these privacy concerns and ensure a secure and enjoyable user experience for our customers.”
What’s Coming Next
With its ability to keep viewers engaged, content personalization/recommendation platforms are helping broadcasters and content distributors retain and grow their audiences today.
As for the future? Taylor expects content recommendations to transcend video and encompass music, gaming and spoken word podcasts.
“As well, content recommendations will incorporate real-time contextual information,” he said. “Factors like time of day, location, current mood, and trending topics will influence content suggestions. And content discovery will extend beyond traditional text-based to include voice-based recommendations, image recognition, and interactive interfaces.”
Artificial intelligence will play a more important role, with broadcasters and content providers working through AI platforms such as ChatGPT to provide recommendations based on users’ questions. “Whether it’s Discord or WhatsApp or Twitch, you can start to create these one-to-one relationships outside of the service providers’ own platforms, leveraging chatbots and generative AI to drive recommendations,” said Riker.
Whatever comes next, the primary goal for content personalization is enhancement, Jones concluded. “While current recommendation engines are better than random selections, there’s room for improvement in targeting content to users’ interests. The challenge lies in moving beyond simply relying on a user’s historical habits.”
James Careless is an award-winning journalist who has written for TV Technology since the 1990s. He has covered HDTV from the days of the six competing HDTV formats that led to the 1993 Grand Alliance, and onwards through ATSC 3.0 and OTT. He also writes for Radio World, along with other publications in aerospace, defense, public safety, streaming media, plus the amusement park industry for something different.