Could AI Become Its Own Worst Enemy?
Arguably, the greatest danger to the future of AI is AI itself
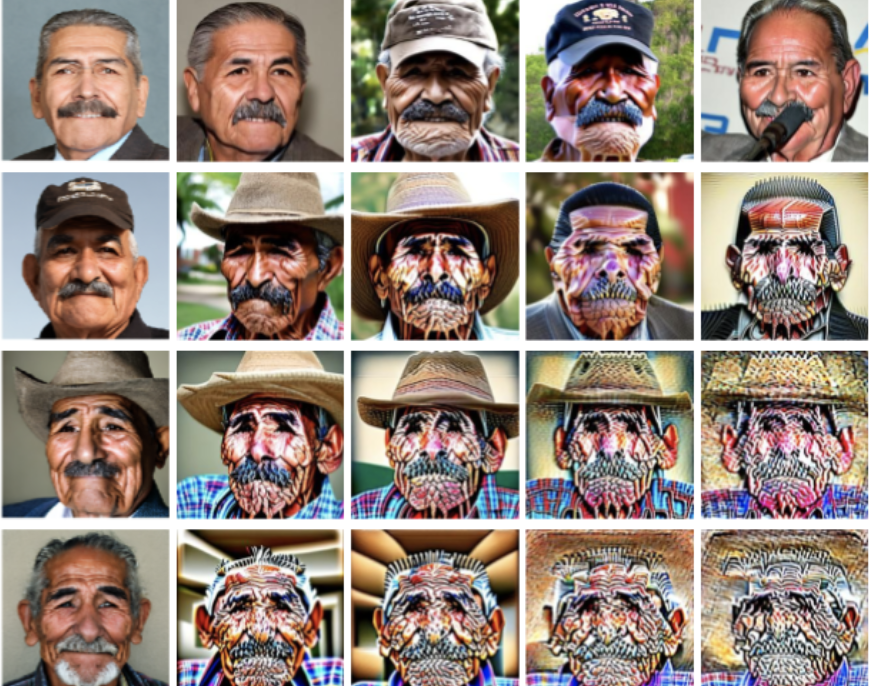
The contemplation of artificial intelligence has a long history, arguably predating the invention of computers. Since the advent of modern computing, the hype cycle for AI has repeated itself numerous times. In 1970, Marvin Minsky, a pioneering figure in AI, was quoted in Life magazine saying, “In from three to eight years we will have a machine with the general intelligence of an average human being.”
This forecast did not materialize then and remains unfulfilled. Once again, the current AI hype cycle is approaching the "trough of disillusionment," but it is expected that we will soon reach a new "plateau of productivity" as the latest advancements are assimilated.
I have been somewhat taken aback by the rapidity with which the present cycle has moved past its peak, leading to more grounded expectations throughout the industry. Over the past couple of years, there has been extensive discussion about the hype and associated fears of AI.
In this column, I aim to delve deeper into the challenges inherent in AI technology itself. Many of these challenges exist independently of their application in media and can arise in any context. Although my focus will be on technological issues, it is crucial to acknowledge substantial non-technical concerns such as economic implications, rights and royalties, cultural transformations, and the legal and regulatory landscape surrounding the technology.
Artificial intelligence has demonstrated its proficiency in handling generic tasks, particularly those with abundant training data. Unfortunately, in creative fields, this often results in subpar content."
Within the scope of technological challenges, there are those where a resolution path is foreseeable and others where no clear solution currently exists. An example of a challenge with a visible path to resolution is electricity usage. We have multiple methods to generate electricity and can eventually develop the necessary infrastructure. Here, however, I will concentrate on a few challenges for which there is currently no evident way to resolve.
When talking about any form of technology, it's crucial to first define the term. In the context of AI, this definition is quite expansive, covering a range of underlying technologies. Commonly, AI now refers predominantly to Generative AI, particularly Large Language Models (LLMs) and associated technologies such as Generative Adversarial Networks (GANs) and others. This article will concentrate specifically on issues related to LLM technology.
Accuracy, Reliability and Quality
By now, we are all aware of some inherent issues in the predictive nature of LLM technology. One of the most prominent concerns is the tendency for these models to "hallucinate," producing results that contain objectively false, bizarre, or highly improbable information. While a certain degree of this can be beneficial, especially in creative tasks, it poses significant challenges in many situations.
Completely avoiding this issue is difficult. Architectures such as RAG (Retrieval Augmented Generation) aim to mitigate this by automatically supplementing the prompt with additional constraining data from traditional data systems like databases. Though this approach is promising, its inconsistent performance makes it difficult to rely on these tools for automated operations. For use cases that demand greater predictability and reliability, it is advisable to use historical automation technologies or trained human operators.
Artificial intelligence has demonstrated its proficiency in handling generic tasks, particularly those with abundant training data. Unfortunately, in creative fields, this often results in subpar content. Most data available to train AI content systems tends to be of low quality and lacks exceptional creativity.
Furthermore, due to the AI's inherent tendency to generate outputs that align with the "average" result, models typically produce quite unremarkable content. To date, AI has not consistently delivered high-quality, creative outputs, and achieving such results seems implausible without human intervention.
Model Collapse
Arguably, the greatest danger to the future of AI is AI itself. Numerous academic studies conducted over the past year have highlighted an apparent irony in our current approach. Essentially, the more effective AI becomes in serving our needs, the less beneficial it ultimately may become. This phenomenon is referred to as model collapse.
As illustrated below, LLMs (Large Language Models) are particularly compelling because they excel at predicting the next likely word, pixel, or other data points in their outputs. These predictions are highly accurate because they tend to average out the possible results. I previously mentioned this issue as a quality concern where the models generally generate "average" content.
A more intrinsic issue emerges from this statistical behavior. If we deploy AI in the real world and the volume of data produced by AI systems increases, the model becomes increasingly skewed by this averaging effect. Paradoxically, the more we use AI effectively, the more challenging it becomes to train it for future utility, leading us towards even more homogenized content.
Even with first-generation models today, you can observe a kind of uniformity in system outputs. As an experiment, try entering 12 different prompts about an elephant or another favorite animal into your preferred image generation platform. Then, use an image search engine to find real-world animal pictures. You'll notice a distinct similarity in the AI-generated images compared to the natural diversity found in actual photos.
The images below illustrate how rapidly these issues arise. After merely five generations of training on datasets containing AI images, the models start producing remarkably similar images. The greater the proportion of AI-generated data within the dataset, the more pronounced this problem becomes.
Introducing AI-generated data into the training set may lead to various other issues, especially if a broader variability is permitted to prevent uniformity. Rare events, like visual artifacts, could become more prevalent and rapidly degrade the dataset in unusual ways, as illustrated by the images below.
I compare the model collapse issue to the problems our industry has faced with analog generational loss. Just as we experienced increased dropouts and image fuzziness with successive generations of tapes, model collapse behaves similarly in its impact.
There are various techniques under discussion to prevent model collapse, but they all present their own challenges. One commonly suggested method is to train exclusively on data that hasn't been generated or influenced by AI. However, if AI effectively serves its purpose, such data will become increasingly scarce. This situation presents a significant irony not lost on this particular human!
Biased Training Data
One of the principal challenges with large language models (LLMs) lies in the inherent bias present in the datasets used for their training. These datasets are frequently sourced from the internet or private data collections. Considering that over 99.999 percent of worldwide information and experiences are neither online nor digitized, these sources introduce a significant bias in training.
The sheer volume of "data" consumed by an individual human daily far exceeds the amount available on the internet. And most of this is neither particularly compelling nor worth preserving and will never be in a form a computer can absorb.
Moreover, the simplest and most apparent realities are seldom directly published on websites, blogs, or other online platforms. Consequently, data is inherently biased towards what we find interesting enough to publish.
A pertinent example is the prevalence of smiling faces in image searches for people. Individuals typically post and store images where they are smiling, which skews the dataset. This bias results in generated images frequently depicting smiling individuals, which does not reflect real-life diversity.
Conclusion
I am inherently optimistic. Despite the challenges I've mentioned, I am enthusiastic about the innovative solutions being tested to address them. It is crucial, however, for media technologists to gain a deeper understanding of the fundamental workings of these technologies to effectively implement them within their organizations.
In my next article, I will explore the essential skillsets we need to develop over the next few years to ensure successful adoption.
Get the TV Tech Newsletter
The professional video industry's #1 source for news, trends and product and tech information. Sign up below.
With more than three decades of M&E experience under his belt, John Footen is a managing director who leads Deloitte Consulting LLP’s media technology and operations practice. He has been a chairperson for various industry technology committees. He earned the SMPTE Medal for Workflow Systems and became a Fellow of SMPTE. He also co-authored a book, called “The Service-Oriented Media Enterprise: SOA, BPM, and Web Services in Professional Media Systems,” and has published many articles in industry publications.