How Multimodal AI is Revolutionizing Content Archiving and Retrieval
Multimodal AI is a type of machine learning that is designed to mimic human perception
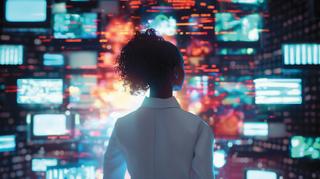
In the digital age, where vast volumes of content are created every second, efficient archiving and retrieval systems are crucial for businesses, researchers, and individuals alike. However, traditional methods often fall short when dealing with the diverse nature of modern content, which includes text, images, audio, and video. With 500+ hours of video uploaded to YouTube alone every minute, it’s virtually impossible to keep pace with the sheer volume of audiovisual content being created and disseminated across today’s multimedia platforms.
Multimodal AI revolutionizes media indexing and search by going beyond simple tags to generate rich, accurate metadata on content. Taking a human approach to media indexing, multimodal AI drastically improves discoverability, enabling media companies to search for precise scenes, shots, and soundbites, accelerating content production workflows.
Key Challenges of Content Archiving and Retrieval
The limitations of traditional content archiving and retrieval systems can drastically impede media companies from finding the hidden gems in their archives. Archived content stored on magnetic tapes presents significant challenges as these tapes and their playback devices are quickly approaching the end of their usable life. As a result, many broadcasters are recognizing the urgent need to digitize their tape archives to preserve their heritage.
Traditional archiving methods encompass manual, logging-by-hand approaches, as well as digital techniques. Manually summarizing and transcribing a one-hour video interview can take up to eight hours. Furthermore, manual archiving is inefficient because human loggers bring unique perspectives, leading to inadvertently biased indexing, overlooked keywords, and sub-par content descriptions. The accuracy and descriptiveness of media files are often influenced by the logger's experience and fatigue levels. Multimodal AI brings a reliable, consistent level of non-biased media asset enrichment.
Leveraging existing metadata can be challenging as well. Inaccuracies on the data sheets left with tapes over the years can create confusion as they're not always precise and don't provide context like AI does.
Performing an archive search with legacy Media Asset Management (MAM) systems is often a cumbersome and ineffective process. Users must enter specific keywords linked to precise metadata tags to achieve relevant results. These results often come as complete files, not short clips, requiring human operators to scroll through extensive footage. Research from Moments Lab shows that up to 50% of a production team’s time can be spent searching for usable content, with some broadcasters reporting that sourcing content for a five-minute video can take up to half a day.
Typically, MAM software is not user-friendly, and content retrieval often falls to one or two “super user” experts within an organization. This situation results in backlogs and missed opportunities to capitalize on archive footage. Additionally, many MAM search functions lack semantic capabilities, making the search experience lacking in nuance and specificity. Users are often left sifting through thousands of irrelevant results.
Why Multimodal AI is a Game Changer
Multimodal AI revolutionizes media archiving and retrieval by understanding and analyzing media content as a human would. Many mainstream AI tools are unimodal, which means that they only process one type of information such as text, audio, or images. Multimodal AI is a type of machine learning that is designed to mimic human perception. Rather than rely on a single data source like unimodal AI, multimodal AI ingests and processes multiple data sources including video, still images, speech, sound, and text to achieve a more detailed and nuanced understanding of media content.
The technology is capable of identifying people, speakers, objects, actions, landmarks, context, shot types, soundbites, and segments. This enables comprehensive media logging and highly intuitive search functions, ultimately allowing media companies to unlock the full potential of their assets. With the decreasing cost of AI and its rapid ability to scan and index hundreds of thousands of media files, media archiving at scale becomes a viable business reality for broadcasters.
Multimodal AI can scan and comprehensively index hundreds of hours of archive footage per minute, providing a true 360-degree view of the content.
One important advantage of multimodal AI is its ability to mitigate biases and hallucinations in generative AI outputs. Developing a confidence score system is as complex as the initial indexing system. Multimodal AI assists in this by leveraging expert systems such as optical character recognition and logo detection to validate generative AI outputs, ensuring greater accuracy and reliability.
Additionally, multimodal AI helps media organizations automate low-value elements of video production workflows, achieve cost savings, and realize new potential sources of revenue.
Multimodal AI can scan and comprehensively index hundreds of hours of archive footage per minute, providing a true 360-degree view of the content. According to research by Moments Lab, media companies can achieve an eightfold increase in productivity for their video ingest teams and save 70% of the time spent on content searches by using multimodal AI. In terms of archive monetization, broadcasters have reported an increase in the marketability of their indexed media assets of up to 50%, with potential annual revenue gains of up to $1 million per 10,000 hours of archived footage. This significant improvement is due to multimodal AI making content more discoverable and reusable.
The discoverability and reusability of media assets heavily depend on the robustness and quality of metadata. Another benefit of multimodal AI is that it ensures the highest quality metadata, thereby enhancing the value and utility of media archives.
Multimodal AI Innovations on the Horizon
Multimodal AI is being used by leading media companies today to unlock the full potential of their digital assets. Future innovations in multimodal technology promise advancements in video analysis and summarization, the creation of a comprehensive world model, deeper understanding of complex videos, and refined sentiment and emotion detection.
Enhanced Video Analysis and Summarization:
Multimodal AI is making significant strides in understanding and analyzing longer videos, making it easier to summarize content even when footage is long, diverse, and detailed. This capability was previously limited, restricting the practical use of multimodal AI on extensive media archives.
The "World Model" Concept:
Researchers are working toward the creation of a "world model" built with multimodal AI, which aims to hold a comprehensive understanding of the world. This model would allow AI to see, hear, read, and understand complex concepts such as physics, human interactions, and movement. Developing this knowledge is challenging but crucial for AI to anticipate and understand video content more accurately. Achieving a world model represents a significant milestone in the AI field.
The development of a world model is a recursive challenge: a good world model aids in understanding videos, but understanding videos is necessary to refine the world model. This creates a race to develop the most advanced video AI that can comprehend the intricacies of the real world.
Complex Video Understanding:
Multimodal AI is evolving to understand more complex videos, including those without sound. This is particularly important for analyzing sports footage, where understanding trajectories and speeds involves complex physics. These advancements will lead to better comprehension and indexing of races, matches, and other sports events.
Sentiment and Emotion Recognition:
Researchers are also focused on improving AI's understanding of human sentiment and emotions. This includes how viewers might perceive a video, such as sitcom footage with a live audience, and how individuals in a video scene are reacting to situations. For example, distinguishing between someone crying and someone laughing based solely on visual cues can be challenging. Multimodal AI must integrate multiple modalities, such as audio and context, to accurately detect and interpret emotions.
These innovations in multimodal AI promise to significantly enhance the capability of AI systems in media analysis, offering deeper insights, more accurate indexing, and a better understanding of complex and lengthy video content.
The Future of Media Content Discovery and Repurposing is Bright With Multimodal AI Multimodal AI transforms content production workflows, uncovers hidden gems, democratizes content discovery, and enables media companies to realize more opportunities to reuse and monetize their media collections, especially through the opportunity to index old and fresh content at scale.
If we consider, as an example, that Moments Lab customers typically use around 600 shots per hour to tell a story, the impact of multimodal AI becomes even more significant. With multimodal AI’s ability to index video content at the shot level, content producers can effortlessly find and retrieve the most compelling moments in their media libraries.
As multimodal AI technology progresses, it will empower media companies to reach higher levels of video production efficiency while opening new revenue streams.
Get the TV Tech Newsletter
The professional video industry's #1 source for news, trends and product and tech information. Sign up below.
James is a skilled sales and management professional with more than 15 years' experience in the broadcast and media industry. James is accomplished in driving business development and securing key partnerships. As Vice President of US Sales at Moments Lab (formerly Newsbridge), James leverages his deep industry knowledge and expertise in AI technologies to manage US operations and achieve notable sales growth. Prior to joining Moments Lab, James refined his abilities in client acquisition, team leadership and strategic market expansion at ES Broadcast and WTS Broadcast.