Machine Learning Drives Artificial Intelligence
But can it go too far?
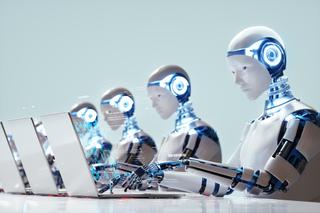
Throughout the 20th century, knowledge has continually expanded, stemming from the evolution of eras such as the industrial revolution, the space program, the atomic-bomb and nuclear energy and, of course, computers. In some cases, it may appear to the masses that artificial intelligence is about as common as a latte or peanut-butter-and-jelly sandwich. Yet the initial developments of AI date at least as far back as the 1950s steadily gaining ground and acceptance through the 1970s.
It wasn’t until the late 1970s and early 1980s that computer science began to emerge from a data-driven industry using large “main-frame” computational systems into platforms for everyday uses at a personal level. While the Mac and early PCs (beginning in the 1980s) were game changers, they were certainly limited on compute power and not designed to “learn” or render complex tasks with modeling or predictive capabilities.
Computers of that time relied on programming based essentially on an “if/then” language structure with simplified core languages aimed at solving repetitive problems driven by human interactions and coordination.
Trial and Error
As sufficient human resources and computer solutions began to develop and create “expert systems” (circa 1990s–2000s) the computer world rapidly moved into a new era—one built around “knowledge” and driven by language models with basic abilities to train itself using repetitive and predictive models not unlike those that infants or toddlers use to hear, absorb, repeat, say and “tune” their mind and physical stature to communicate, to learn physical principles (such as walking), coordination and more.
If you’ve ever observed a two-year old learn how to maneuver around a slick pool edge surface, make their way to the steps into a pool and gradually ease themselves into the water in a fashion that uses mental observation (aka “memory senses”), trial and error, and repetitive steps (slow walking or crawling) while a parent also observes the child’s actions to mitigate accidents or errors—you’ll quickly see, figuratively, what occurs in a set of computers or servers built to use software to train itself over time to create in part what will become a large language model (LLM) by applying its programming repetitively that eventually finds a way (i.e., to model) the original programming into a formidable model that reaches the desired goal.
Without Explicit Programming
Machine learning is just that kind of process and is the basis of AI, whereby computers can learn without being explicitly programmed. This generalization of ML has classifications that are utilized to differing degrees as diagrammed in the figure on Machine Learning Tasks (Fig. 1). Fundamentally, machine learning involves feeding data into coding algorithms that can then be trained (through repetition with large sums of data) to identify patterns and make predictions, which then form new data sets from which to better predict the appropriate outcome or solution.
A variety of applications such as image and speech recognition, natural language processing and recommendation platforms make up a new library of systems. The value relationship gained in this training process can be developed by (T) defining a task; (P) establishing a performance figure or criteria (i.e., how well did it do or how far off were the results); and (E) finding a resulting data set, the experience, given the data set it was provided for such an analysis.
Machine learning is a continual process whereby trials create results that get closer and closer to the “right solution” through reinforcement. Computers/servers learn which data set is then classified as “more right” or “less right” (i.e., more or less correct); and then stores those results that then modify the learning algorithm(s) until the practice gets as close to “fully right” as possible without “overshooting” the answer and risking a data overload or a false (hallucinogenic) outcome.
One downfall in ML is that the system may go “too far” (i.e., it has too many iterations), which then generates an exaggerated or wrong output and produces a “false-positive” that gets further from the proper or needed solution. Then one questions, “just how far does the generative process go before it is stopped?” When the system gets to the “regression” point—a case where the outputs are continuous rather than individual or discrete—then a categorizing sensing algorithm would necessitate supervisory termination or a resteering of the operation to a more dimensioned (contained) level, which restricts the continued processing.
So, in addition to the learning algorithm, there are sets of management algorithms that must be applied throughout the learning process to mitigate these so called “hallucination” possibilities. Remember the toddler in the pool, this manager may be the parent in this case, the individual who stops the child from being hurt or risking a task (T) that could be catastrophic in nature.
Buzzwords such as "machine learning," "deep learning" and "artificial intelligence" have many people thinking these are all the same thing whenever they hear the phrase “AI.”"
ML can (and is) used in many everyday solutions including email filtering, telephone SPAM filtering, anomaly detection in financial institutions, social media facial recognition, customer data analysis (purchase history, demographics) and trends, price adjustments (such as with non-incognito searching), and emerging advanced solutions including self-driving cars and medical diagnostics. The “balancing” apparatus must weigh multiple solutions, alternatives and decision points, which in turn keep a runaway situation from occurring, resulting in an unnatural or impossible situation or solution.
Industry Challenges-Bias & Fairness
Besides the rapidly developing capabilities, there are as many challenges in this evolving AI industry as there are opportunities. Data Bias and Fairness (e.g., in social media) is highly dependent on the data it has available for training. Bias can obviously lean toward and potentially lend to discriminatory solutions.
Privacy protection as well as security breaches head the users into areas that result in illegal or illegitimate practices. Given the ease in spinning up huge data server systems in the cloud, the possibility of running tens of thousands of iterations on passwords or account numbers means the risk to the customer (as in credit card fraud) can grow exponentially to the number of credit card holders. Banks and credit services use very complex AI models to protect their customers.
This in turn opens the door to another level of AI—that is risk, fraud protection analysis and monitoring. It’s a huge cost to the credit card companies, but one that must be spent in order to protect their integrity.
Another concern is in automation and the potential for job displacement. It is inevitable that some people will be displaced by automated AI solutions. In turn, these new dimensions are opening up new job opportunities across new sectors of the workforce, such as data analysis, machine learning management, data visualization, cloud tool development, laboratory assistants or managers who test and hone these algorithms.
There continue to be many misconceptions related to these new words and their actions. Buzzwords such as "machine learning," "deep learning" and "artificial intelligence" have many people thinking these are all the same thing whenever they hear the phrase “AI.” Regulations are being developed internationally and within our own legislatures that directly relate the “AI word” to machine learning or vice versa.
Most of these early “rules” are done in a pacifistic way, likely because the legislative authors have little actual knowledge or background in this area. Obviously, we risk going in the negative direction by reacting improperly or too rapidly—but things will surely happen that will need to be corrected further downstream. Doing nothing can be as risky as doing too much.
Easily Defined and Managed
As for the media and entertainment industry, efforts are well underway to put dimension on the topics of AI, ML and such. As with any of the previous standards developed, user inputs and user requirements become the foundation for the path towards a standardization process. We start with definitions that are crafted to applications, then refine the definitions that reinforce repeatable and useful applications. Through generous feedback and group participation, committee efforts put brackets around the fragments of the structures to the point that the systems can be managed easily, effectively and consistently.
For example, industry might use AI or ML to assemble, test or refine structures, which will in turn allow others to learn the proper and appropriate uses of this relatively new technology. Guidance is important, as such, SMPTE, through the development of Engineering Report (ER) 1010:2023 “Artificial Intelligence and Media,” is moving forward on this development and has a usable structure from which newbies and experienced personnel can gain value from.
Even the news media has interest in its future in AI. In their book “Artificial Intelligence in News Media: Current Perceptions and Future Outlook,” Mathias-Felipe de-Lima-Santos and Wilson Ceron state that “news media has been greatly disrupted by the potential of technologically driven approaches in the creation, production and distribution of news products and services.” l
Get the TV Tech Newsletter
The professional video industry's #1 source for news, trends and product and tech information. Sign up below.
Karl Paulsen recently retired as a CTO and has regularly contributed to TV Tech on topics related to media, networking, workflow, cloud and systemization for the media and entertainment industry. He is a SMPTE Fellow with more than 50 years of engineering and managerial experience in commercial TV and radio broadcasting. For over 25 years he has written on featured topics in TV Tech magazine—penning the magazine’s “Storage and Media Technologies” and “Cloudspotter’s Journal” columns.