Uncorking AI
Separating fact from fiction about the defining technology of our time
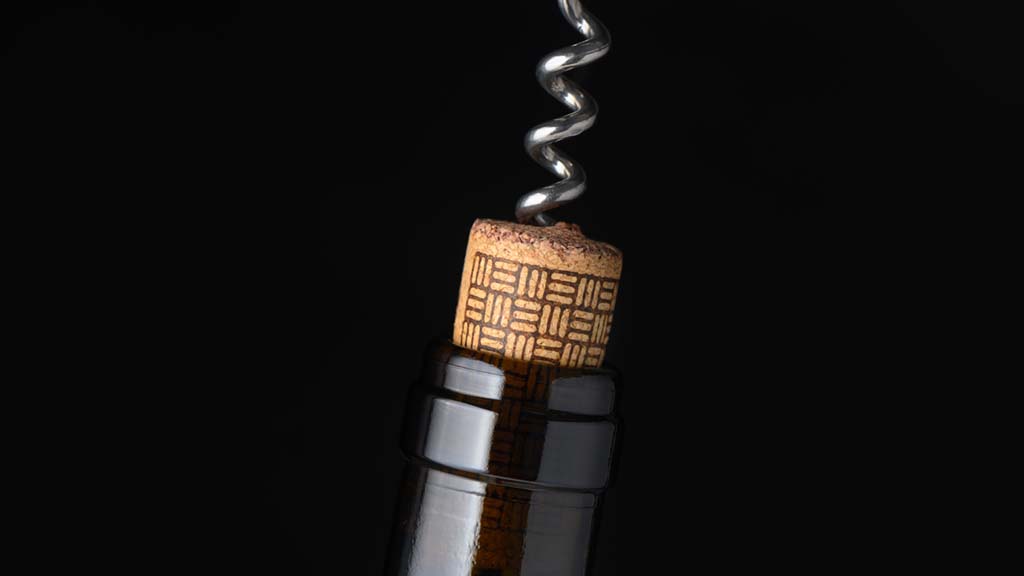
Using artificial intelligence (AI) for practical purposes is gradually making it into the mainstream public world, despite the fact that it has been in regular use for many “business & industry” purposes for probably a decade. While the media seems to be adding the term “AI” as the resolute action for many things such as graphics, text creation, false or misleading anything, sadly AI is getting a bad wrap in many circles.
This may, indeed, be because AI is so misunderstood—which, frankly, is why my TV Tech colleague John Footen and I created these monthly columns on this rapidly advancing and maturing technology.
This month—in the first of two parts—I’m going to go back to some fundamentals about what it takes to use AI (specifically “generative AI”) by providing some overviews graphically and textually to kick-start “what you need to know about AI” to uncork the genie into practical use and applications. We need to go back a few years and start to interleave other sets of terms to place the bigger picture into a better perspective.
For the First Time in Forever
For the first time, we can now actually “ask data a question” in human speak/text and get an “original” answer as complete content, returned in human text as speech or as a visual interaction. However, these changes are not without a price—in power, real estate and infrastructure change. Some examples follow.
For perspective, “a single Google search can power a 100-watt lightbulb for 11 seconds,” says Bill Kleyman, CEO of Apolo, an AI platform and infrastructure company. When you shift to new AI solutions like ChatGPT, this multiplies by many fold—which means the computer is 600-800x more powerful than Google search, leading to as much as a 600-800-power draw increase.
• Game theory and AI, human behavior, evolutionary dynamics, health data science;
• Biomedical informatics, machine learning, AI for healthcare, medical imaging, digital pathology;
• Video understanding, computer vision, machine learning, multimodal learning, cognitive science;
• Human-AI interaction, computational health, data science, natural language processing, conversational assistants, deep learning;
• Complex systems, machine learning, networks, computational harmonic analysis;
• Ethical AI; AI and society; large language models (“LLMs”); generative AI (“gen AI bots”); natural language processing; computational social science;
• Graph/data mining, graph neural networks, interpretable machine learning, program understanding and synthesis, and
• 3D point clouds, statistical physics, loss landscape, neural-network weight analysis, information theory, coded computing—diagnosing and mitigating failures in machine learning models.
Thus, it takes a lot of data to get AI to function, which is why we hear that the prominent players (Google, Oracle, Amazon, Microsoft, etc.) are building gigantic warehouse-like data centers—the scale of multiple sport stadiums—in areas where megawatts of power are available and land is spacious enough for extended growth well into the future.
Big Data
You’ve likely heard the term “big data” for quite some time but only wondered what it really means or how the term is applied. In the early days, not many realized the value and importance of “big data” to the concepts and principles of AI. Even today, the mention of “big data” is not applied much when speaking about AI in the general sense. But without “big data” and its associated corollaries (see the glossary sidebar)—we could not have effective, consistent, believable, and trustworthy AI.
AI needs lots of data to train its systems on, which is how large language models leverage massive data collections to build a “model” on which to train the systems. An LLM will be used to generate a “consensus” from which to output an answer, but this takes much more in infrastructure than what we’ve seen in the past.
Power Density
According to the 2024 AFCOM data center report and presentations “Key-Trends-and-Technologies-Impacting-Data-Centers-in-2024-and-Beyond,” based on data from 2021, a datacenter rackhousing “IT-centric” equipment averaged around 7kW per rack; but we have already arrived at average rack densities of 12 kW equipment.
For an AI complex, that is nowhere near enough power to contain the kinds and types of compute systems that AI demands. According to the report, “the upward trajectory to [will] continue with 20 kW averages likely by 2030.”
Landscape
The Generative AI (GenAI) application landscape is a moving target, constantly growing and shifting across all industries but specifically in areas relative to: (a) imaging, (b) video; (c) text; (d) research; (e) 3D: modeling/manufacturing and medical; (f) speech: translation; and (g) code: i.e., documentation, web app building, text to SQL (like) databased, actual self-created code-generation. Fig 1 in the sidebar outlines areas where AI is being applied in everything from research to manufacturing to communications.
AI Capabilities
GenAI can be used to alter the “tone” of a segment of content, based on the audience it is to address. In essence, by altering the phrasing and the content, the text, visual details or audio can be adjusted (slanted or enhanced) to fit the audience it is being supplied to. Such alterations may include “setting your voice” i.e., adjusting your generated text to sound. Prompts may include (a) suggest and construct counterarguments, (b) improve the emphasis on a certain section, or (c) to regenerate new text for an audience that has not previously used a new or differing experiential design.
Web design is one which can be dynamically changed based upon the “user experience” or by leveraging the data secured from previous searches that the web user has looked at or searched for. This is very common on search engines whereby the user is “pushed” into sponsored areas of a document (webpage) that would be attractive to the user and promotional for the advertiser.
Heretofore, this was not possible without GenAI technology, but now features such as “intuitive navigation” or “responsive design” or “visual hierarchy” which include new “interactive elements” (Fig. 1), can be used tp automatically generate and create “user-specific” pages that fit the both the sponsor/advertiser objectives and the expected needs of that user. This is an important part of how AI is pushed into modern media.
Visualization
Visual hierarchy is a technique whereby graphic elements are resized or adjusted to drive the user’s attention to more important elements on a webpage. By capturing user data from previous searches or by leveraging “big data” from a machine learning process, the tone of the content may be “swayed” to best fit the specific user-type or perspective.
Gen AI is used to make the user “want” to look deeper or find a media element more pleasing—including altering the audio (musical tones, speed, intensity, softness, etc.,) or speeding up or better emphasize the verbal rates of the text or by creating art which is typically desired (or found) by similar users as a particular type of said art or video content.
This became more emphasized by changes which the original owners of the 007-franchises, whose IP is now owned by Amazon. One of the expected “values” to be created by Amazon will be the use of GenAI technologies to create new stories, new content, and/or the selection of new talent who will attract more and consistent “Bond-Enthusiasts.” All of this, despite Amazon’s CEO Andy Jassy’s denials that “he does not envision that AI is going to write a Bond movie any time soon.”
Greater Depth
By continuing to improve or elaborate on the content, users may then add more questions or comments to those replies created by the prompts. However, one should be cautious: Users could “overcompensate” the GenAI bot replies with too many follow-up inquiries. The results could be what is known as “hallucinations,” whereby the bot begins to make up artificial answers which may go off-base in both topic and reaction.
Users will then learn just how far to push the GenAI bot, and where the answer has received enough iteration or depth to “call it quits.” This is one of the areas where researchers are worried that much greater time with AI will be necessary for complete confidence to be achieved.
Watch for the conclusion of this topic in the September issue of TV Tech.
The professional video industry's #1 source for news, trends and product and tech information. Sign up below.
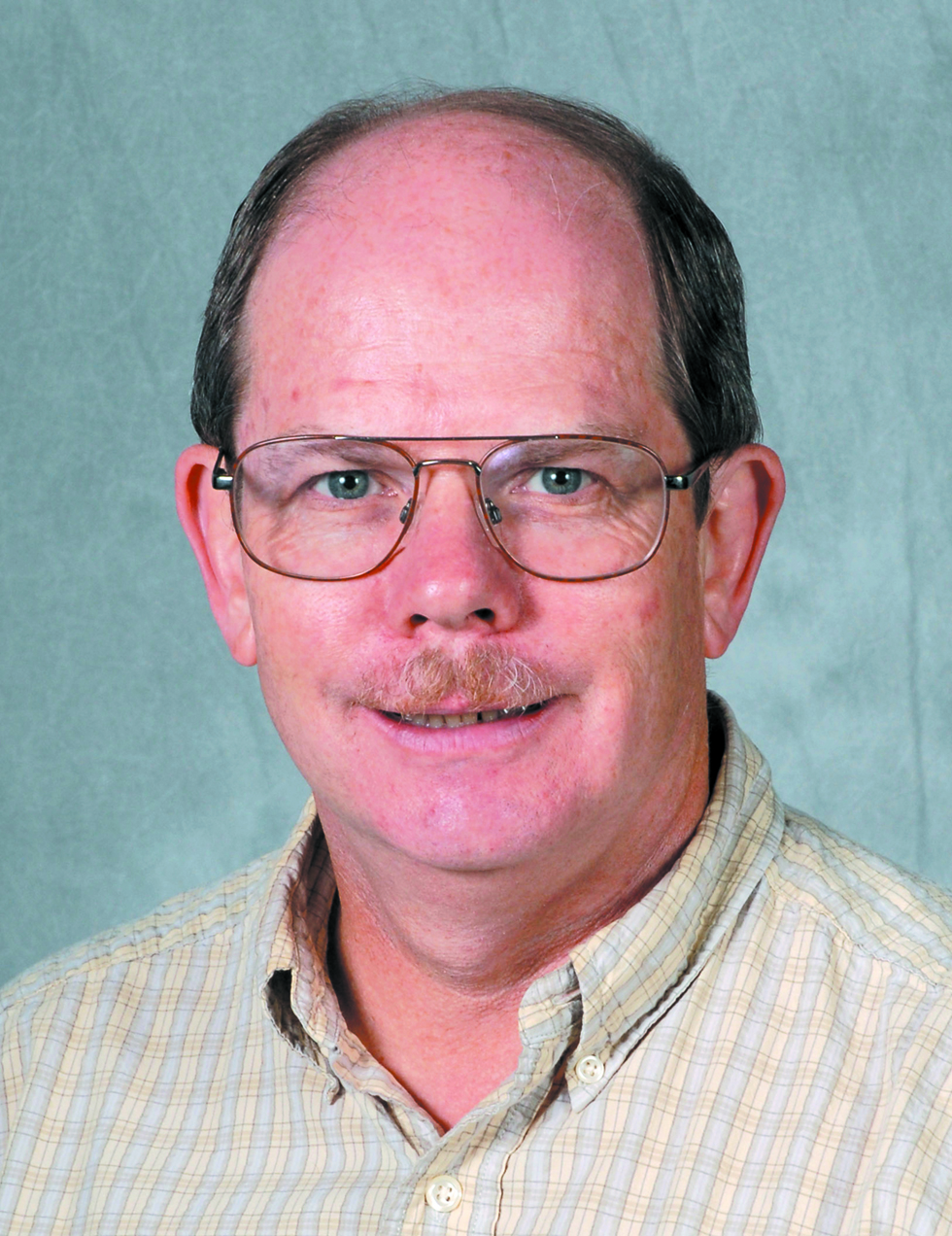
Karl Paulsen recently retired as a CTO and has regularly contributed to TV Tech on topics related to media, networking, workflow, cloud and systemization for the media and entertainment industry. He is a SMPTE Fellow with more than 50 years of engineering and managerial experience in commercial TV and radio broadcasting. For over 25 years he has written on featured topics in TV Tech magazine—penning the magazine’s “Storage and Media Technologies” and “Cloudspotter’s Journal” columns.