Unlocking AI Potential for Media Organizations
Integrating artificial intelligence empowers media companies to streamline production, improve content distribution and enhance audience engagement through personalization
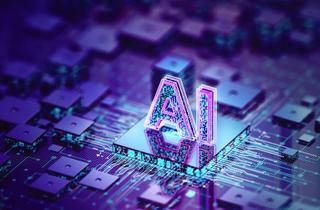
As artificial intelligence (AI) matures, media companies are under increasing pressure to integrate it effectively or risk falling behind in a rapidly evolving digital landscape. AI is reshaping the media industry, enhancing everything from content personalization to advertising strategies and operational efficiencies. However, the implementation of AI is complex, with risks of misalignment, high costs and operational disruptions. For media organizations, the critical question is not whether to adopt AI, but how to do so in a manner that delivers measurable value.
Integrating AI empowers media companies to streamline production, improve content distribution and enhance audience engagement through personalization. Yet, each AI application presents unique demands and challenges, depending on the specific use cases and organizational context. A structured approach to integration is essential for harnessing AI’s potential while avoiding common pitfalls.
Assessing AI Use Cases
Successful AI integration begins with assessing the organization’s readiness for each use case. Broader AI applications that affect multiple departments and data sources require thorough evaluations to ensure alignment with business priorities. In contrast, narrower use cases, such as improving encoder performance, are typically easier to assess and may require simpler evaluation models.
Successful AI integration begins with assessing the organization’s readiness for each use case.”
Narayanan Rajan
Next, businesses need to clearly define desired outcomes tied to operational KPIs, such as cost savings, customer engagement, and revenue growth. These benchmarks provide a measurable basis for gauging success. Finally, it is important to evaluate the robustness of available solutions and identify performance thresholds for success. Since most organizations leverage third-party AI models accessed via APIs, understanding expected results through straightforward metrics—like quality, delivery speed and bandwidth utilization—is crucial. These steps ensure that selected AI use cases deliver practical, measurable value.
Key Considerations for AI Integration
There are a few factors that need to be considered when evaluating an AI solution for your business. Below is an example evaluation model for assessing whether or not to integrate AI into an encoding workflow:
- Total Cost of Ownership (TCO): TCO is critical in evaluating AI tools, encompassing all costs associated with AI, including computational resources and software licensing fees. For instance, while AI can reduce bandwidth costs in encoding workflows, it may also increase demand for computational resources. Organizations should benchmark current costs and compare them to potential AI-powered solutions to maintain a balanced view of costs and savings. It’s essential to recognize that AI processes evolve, meaning TCO can change as new, more efficient versions are released.
- End User Impact: Evaluating end-user impact is vital—for example, AI processing in encoding can introduce latency, and can be particularly disruptive for live-streamed content. The evaluation should include thresholds for acceptable latency and consider potential client-side effects. It’s crucial to ensure that AI enhancements do not compromise viewer experience, especially in real-time applications where delays can lead to churn and reduced engagement.
- Operational Impact: AI integration often necessitates operational changes, including new monitoring processes, staff retraining, and sustainability considerations. For example, AI-powered encoding may require ongoing monitoring to maintain picture quality and optimize bandwidth savings. Staff may need training on updated performance metrics or configurations. Additionally, evaluating the sustainability implications of increased computational loads is important, as it can impact the organization’s ESG (Environmental, Social, Governance) commitments.
- Systemic Risks: AI systems often interact with other automated workflows, making it crucial to understand systemic risks. Cascading errors could arise if one AI-enabled system malfunctions and affects others downstream. To mitigate these risks, companies should conduct thorough testing, ideally simulating failures within test environments to ensure robust failsafes and redundancies. Properly understanding and managing these risks helps avoid disruptions and maintain the stability of end-to-end workflows.
- Ethical and Privacy Considerations: Ethical and privacy concerns are critical in any AI evaluation framework. Companies must assess whether an AI system could unintentionally alter content or access sensitive customer data. For example, an AI-enabled encoding system might support in-frame brand detection for ad monetization, raising questions about content integrity and creator rights. It’s essential to ensure that all controls and permissions comply with ethical standards and privacy regulations for responsible AI implementation.
To implement AI effectively, organizations should initiate pilot programs in controlled environments. These pilot tests allow for performance assessments and tool refinements before broader deployment. For instance, the BBC has successfully tested AI-driven content personalization, demonstrating the importance of incorporating rollback mechanisms to mitigate risks.
Additionally, fostering a culture of experimentation is crucial. Non-production trials enable companies to evaluate AI benefits without disrupting operations, with 2023 Gartner data indicating that nearly half of executives are currently testing generative AI.
By assessing readiness for specific use cases, clearly defining measurable outcomes, and evaluating costs alongside ethical implications, organizations can navigate the complexities of AI adoption effectively. A well-structured framework not only mitigates risks but also ensures that AI implementations deliver tangible value. Embracing a culture of experimentation will further enable companies to adapt to an evolving landscape, positioning them for sustained success in a competitive market.
Get the TV Tech Newsletter
The professional video industry's #1 source for news, trends and product and tech information. Sign up below.
With over 20 years of executive experience in the media and telecoms space including TandbergTV, Ericsson, and Mediakind, Narayanan Rajan has led transformation and integration initiatives in engineering and operations roles across multiple organizations. As CEO of Media Excel, he now leads an organization developing cutting-edge technology for encoding and transcoding, including AI-based enhancements to improve encoding performance.